User Engagement and Scientific Research
Program Areas – Science and Technology Policy, Complex Socio-technical Systems
A Typology for Assessing the Role of Users in Scientific Research
Elizabeth C. McNie, Adam Parris, Dan Sarewitz
I Overview
Decision makers call upon and fund science to help clarify and resolve many types of problems (OECD 2002; America COMPETES Act 2007; Bush 1945). They expect research to create useful information to help inform solutions to intractable problems, catalyze innovation, and provide information that not only educates stakeholders, but also expand alternatives, clarify choices, and aid in formulating and implementing policy decisions (Dilling and Lemos 2011; Sarewitz and Pielke 2007).
But linking science with decision making to help solve problems is challenging. Often when responding to such problems we simply produce more science, and not necessarily “the right science” (NRC 2005, 2009). Intended users of the scientific information may be unaware that it exists, or be unable to use what is available. The difficulty of actively linking the supply of scientific information with users’ demands leads to missed opportunities for science to better inform policy (see Table 1; Sarewitz and Pielke 2007). Such “missed opportunities” occur for many reasons. Here we are concerned with the tendency to view and assess research in isolation from the context of its use, and simply in terms of whether it is “basic” or “applied.” Such science-centric approaches have great value in producing new knowledge, but are inadequate to address the growing complexity of problems typically facing decision makers, and may in fact simply reinforce a structural gap between the “production and use of scientific information” (Kirchhoff et al. 2013, p. 407).
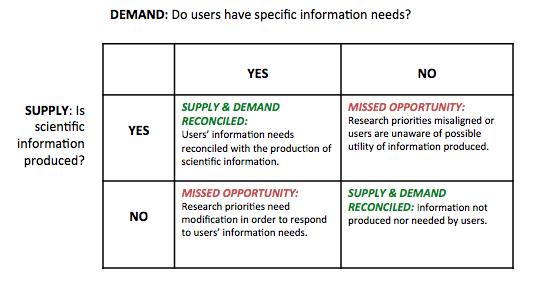
Table 1: Missed Opportunity Matrix
Over two dozen different terms describing scientific research have been described or adopted by the National Science Foundation, National Science Board, Office of Management and Budget, Organization for Economic Cooperation and Development, and science-policy researchers and others during this century and the last (see Table 2). Research types have been defined by many variables, although the differences between many types are often minor or semantic (Stokes 1997; Calvert 2006). Nonetheless the research categories of “basic” and “applied” remain the epistemic norms in the science community, and as a result, most research types are classified as one or the other, and distinguished primarily by two overarching criteria: the motivation for research (fundamental discovery vs. application of knowledge) and temporal delay to application of research results (from a few years to decades) (NSB 2010; OECD 2002). Despite many efforts to refine research typologies, none of the types considered and adopted by national or international science policy bodies identifies the role of users in the shaping of research, and none of the types adequately address the need to reconcile the production of scientific information with the context in which such information is used. In fact the OECD even goes as far to disqualify from consideration as basic or applied research any activities or personnel that are actively engaged with potential users (2002).
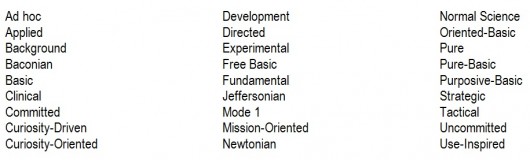
Table 2: Common Research Types
Many practitioners and scholars of science policy have come to recognize that the basic/applied typology may conceal as much as it reveals. For example, in Stokes’ well-known conception of use-inspired research, he added to the standard dichotomy a new dimension (Pasteur’s Quadrant) that accommodated the recognition that research, whether basic or applied, is commonly influenced by considerations of use (1997). Little to no progress has been made in translating such insights into criteria for research design,[1] and even Stokes failed to consider the role of users themselves. Our perspective here is that the character of scientific knowledge, the intended use of science, and the role of users in the research process will often be directly pertinent to appropriate research design.
Some have suggested that directing research toward specific outcomes is unproductive, may limit scientists’ exploration of possible alternative solutions (Merton 1945), may lead to concerns over scientific accountability (Lövbrand 2011), and may even be harmful to science (Polanyi 1962). Such concerns in total question whether science can be shaped, let alone shaped by users. But extensive research has shown that science is a social process influenced by societal and individual values and norms (Latour 1987; Jasanoff 2004; van den Hove 2007) and is therefore amenable to being shaped and informed by scientists and users alike (as well by as other factors such as institutional culture and professional incentives). Directing research toward practical ends has always been a part of our scientific enterprise, and doing so need not drive out fundamental and novel discoveries (Stokes 1997; Logar 2009; McNie 2008). Increasingly, the scientific community itself is coming to recognize the need for new science institutions that better support, incentivize, and train scientists to perform research to meet societal needs (Averyt 2010; Knapp and Trainor 2013; NRC 2009; NRC 2010). To do that, we need better approaches to view, deliberate on, shape, implement and evaluate research. The typology presented here has been developed as a tool for supporting such approaches. It is intended for use especially by science program managers and designers, although we anticipate that it will be modified, adapted, and improved both through use by practitioners and through further scholarly analysis.
II An Expanded Typology
This paper introduces a new, multi-dimensional research typology that can describe different research types in order to improve our understanding of research in general, help inform research design, and assess the organizational and institutional resources needed to manage different types of research. Application of this typology may help improve science-policy decisions by revealing the ways in which science programs may or may not be appropriately reconciled with the problem context they are supposed to address.
One consideration that cuts across the typology is the role of the knowledge user. “Users” constitute a broad category that includes people or organizations that bring the results of research into decision making contexts and activities. But users also engage with researchers at various times and venues in the research process, and this engagement itself influences research and its value for users. As well, users may need or benefit from different kinds of information at different points in any decision process. For example, in the early phases of decision making, users may need to simply improve their understanding of the problem, whereas in later phases of decision making users may need highly context-specific information. Given the continuum of decision making, users themselves may change as the problem or context for action evolves. Users may be program managers, the media or other science communicators, educators, policy makers, elected officials, interested citizens, and more. Other researchers or technologists may also be users. Given such variety, it is important to understand and articulate who the intended users of the research outputs are, as this will help inform and characterize the research activity.
Underlying this discussion of users are questions of influence: Who gets to influence the design, implementation, and use of research? What is the extent of that influence? The distribution of influence among unique users is not uniform and varies by social, political, economic, intellectual, and cultural dimensions.
The typology divides research by three general activities, each of which is subdivided into more specific attributes. Knowledge Production describes attributes such as the nature of expertise, goals of research, and treatment of uncertainty. Learning and Engagement describes attributes such as what is learned, how knowledge is exchanged with users, who participates in the exchange network, and the role of social capital in transferring knowledge. Organizational and Institutional Processes describes attributes such as research outputs, human capital, institutional accessibility, organizational flexibility, boundary work, and approaches for evaluation, which together help inform how resources can be identified and deployed to support research. The attributes are situated on an idealized spectrum bound by value-laden criteria ranging from strongly science-centric to strongly user-oriented (see Figure 1). The left side of each spectrum represents research criteria focused on achieving ends internal to science—what we term “science values” (Meyer 2011). This research tends to be more disconnected from explicit consideration of the context of use and involvement of users, that is, science is treated as a closed system. The right side represents research criteria focused on achieving ends external to the research itself—what we term “user values.” Such research is open to engagement with actors other than the core researchers themselves and is justified by the expectation of providing information and knowledge for various users and uses. A research activity is characterized in full by assessing where it lies on the spectrum of value criteria for the entire suite of attributes.
III Research Activities
In this section we briefly describe and define each element of the typology. The typology is divided into three organizing categories (columns in Figure 1): Activity, Attribute, and Spectra of Research Criteria. The Activity column separates the research endeavor into major subcomponents; the Attribute column identifies the key sub-categories of each Activity; and the Spectra of Research Criteria column shows, for each Attribute, a pair of evaluative criteria that define a spectrum along which any given research program can be qualitatively located. For example, in considering Knowledge Production, approaches to addressing the attribute of Uncertainty may range along a spectrum from those aimed at reducing uncertainty to those aimed at managing it. Of course, most research activities lie between the extremes. They typology allows this intermediate domain to be explored and characterized with attention to any or all of the fifteen research attributes. We intend it to be a heuristic device that can help inform and improve science-policy decisions.
Activity 1: Knowledge Production
Knowledge Production addresses what is credible, who is credible, and what ways of creating knowledge are credible (Epstein 1995). Knowledge Production consists not just of facts but includes tacit skills and the wisdom of lived experiences, while acknowledging that freedom from bias and social influence is never entirely possible (Jasanoff 2004; Latour 1987; Latour and Woolgar 1979).
1. Knowledge Production: Attributes and Spectra of Research Criteria
A. Expertise:
Who has the credibility to produce knowledge?
Epistemic: Experts have specific norms and behaviors consistent with their epistemic communities. Expertise tends to be oriented by discipline and is established by attainment of a Ph.D.
Experiential: Expertise expands from disciplines to include policy, economic, bureaucratic, community, and lay expertise, each of which comes with its own norms and criteria for quality. Expertise also includes lived experiences and proximity to the problem.
B. Relevance:
What is the source of relevance to solving the specific problem?
General: Research is oriented toward developing and testing hypotheses with the aim of informing theories. Consequently, outputs from research tend toward global instead of local scales and are broadly relevant. Significant time delay between research and application also reduces relevance.
Contextual: Research is oriented toward producing knowledge that focuses on spatial and temporal scales specific to various dimensions of a pre-identified decision. Solving discrete problems requires that information be context-sensitive and needs to consider the appropriate physical, social, and natural scales.
C. Disciplinary Focus:
How discipline-driven are the knowledge production activities?
Singular, Narrow: Research is largely guided by single or sub-disciplines. Knowledge is often characterized by a reductionist worldview in which systems are divided into smaller parts and analyzed in isolation, and is guided by problem formulation rooted in disciplines. Interdisciplinary research may also occur, but research questions are still largely informed by a reductionist worldview and organized around problems that are defined scientifically.
Transdisciplinary, Diverse: Research is transdisciplinary, that is, is organized around problems that are defined by the context of use, and will often incorporate social, physical, and natural sciences. Such research is necessary, for example, when knowledge is sought to inform decisions related to coupled human-environmental systems (Clark and Dickson 2003; Berkes 2009; Ziegler and Ott 2011).
D. Uncertainty:
How do researchers understand and address the problem of uncertainty in knowledge production?
Reduce: Uncertainty and statistical errors are to be reduced as much as possible, while simultaneously ensuring the highest degree of accuracy and precision.
Manage: In some problems uncertainty is irreducible and must be managed as an accepted condition of more complex and interconnected realities (van den Hove 2007; Funtowicz and Ravetz 1993). More information does not necessarily reduce uncertainty and can in fact increase it (Sarewitz 2004). Reducing uncertainties, often considered essential for policy, is not necessarily a pre-condition for making robust decisions that reduce vulnerabilities (Lempert et al. 2004).
E. Goals for Research:
Is the knowledge produced to provide insights into science itself, or into questions and problems outside of science?
Exploratory: Research is driven by curiosity and not constrained by specific goals. If diffuse goals are informing research questions, application of knowledge may be years or decades away.
Outcomes-oriented: Research is shaped by those people who will use the knowledge. Use may involve expanding understanding of a discrete problem or may feed into specific decisions, policies, plans, etc.
Activity 2: Learning and Engagement
Learning requires information and a process of transformation in which behavior, knowledge, skills, etc. are developed or changed (Knowles et al. 1998; Mezirow 1997). Social learning is contextual and iterative (Pahl-Wostl 2009) and requires systems thinking, communication, and negotiation (Keen and Mahatny 2006). Learning becomes more difficult as problems become less structured and more complex (Argyris 1976).
2. Learning and Engagement: Attributes and Spectra of Research Criteria
A. Learning:
In what ways do the research outputs change the knowledge or decision-making system?
Theoretical: Learning is focused on understanding theories and focuses on the absorption of explicit knowledge that can be easily transferred between people through documents, patents, and procedures.
Social, Practical: Learning is about understanding new things, but is also focused on new techniques, better approaches, and developing new policies, plans, and behavior by exchanging tacit knowledge that is difficult to codify, takes time to explain and learn, and is embedded in relationships (Nonaka 1994).
B. Knowledge Exchange (a process of “generating, sharing, and/or using knowledge through various methods appropriate to the context, purpose, and participants involved” [Fazey et al. 2013, p. 19]):
To what extent, and how, is knowledge exchanged?
Restricted, Linear: Exchange is limited primarily to within the researchers’ own epistemic community and occasionally to the general public through the form of press releases or news articles. If it occurs, communication is one-way from science to society.
Iterative, Influential: Methods can include knowledge brokering, informing, consulting, collaborating, mediating, and negotiating, among others (Michaels 2009; Lemos et al. 2012), and leads to more “novel forms of the contextualization of knowledge” (Nowotny et al. 2002, p. 206). Two-way, iterative or “multi-way” communication is needed for the production of useful information to inform decisions (Lemos and Morehouse 2005; Kirchhoff et al. 2013). Knowledge may need to be “brokered” by individuals or organizations that are trained or designed to do this work (Guston 2001; Clark et al. 2011). Brokering is fundamentally about building relationships between the different actors (hence the need for social capital) and leveraging knowledge networks.
C. Network Participation:
Who participates in the knowledge network?
Homogeneous: Participation in research is limited to other researchers or sometimes to a single researcher. Users or the public are not included in the shaping of research agendas.
Heterogeneous: Users play a moderate to major role in the shaping of research agendas. Participants may include researchers from multiple disciplines, as well as policy, economic, bureaucratic and lay experts. The researcher network is larger, spanning multiple scales (e.g. local to global).
D. Social Capital (relationships and “goodwill that others have toward us,” the effects of which flow from the “information, influence, and solidarity such goodwill makes available” [Adler and Kwon 2002, p. 18]):
How important is the development and deployment of social capital?
Negligible: The need for creating or deploying social capital may be non-existent or exist only within the researchers’ epistemic community.
Significant: Social capital, trust, and relationships are necessary to create and share knowledge (Levin and Cross 2004). People are more willing to share useful information, listen, and absorb knowledge when the relationship is grounded in trust (Levin and Cross 2004; Lemos et al. 2012).
Activity 3: Organizational and Institutional Processes
The organization of work, research, incentives, and both formal and informal rules, all shape the process of work, knowledge production, and interactions between groups (Trist 1981; Geels 2004). Research processes and organizations are also subject to the same socio-technical considerations as other forms of work, albeit with different characteristics, norms, identities, and processes (Jasanoff 2004).
3. Organizational and Institutional Processes: Attributes and Spectra of Research Criteria
A. Accessibility:
How accessible to users are the researchers and their organizations or institutions?
Constrained: Research organizations and researchers are often difficult to access due to physical constraints (location of researchers or their organization) or institutional constraints (placement of the research organization within other organizations or institutions), making it difficult for users to gain access.
High: Researchers and their organizations are located proximate to the user and problem. Organizations are often designed to facilitate easy access to researchers and knowledge resources. Access by users is prioritized in organizational activities.
B. Outputs:
How various are the research outputs?
Narrow: Outputs are directed toward a limited audience. The most common output is the peer-reviewed publication, followed by reports, patents, conference attendance, new methods and processes, workshops, etc.
Diverse: These approaches yield similar outputs to research disconnected from users’ needs, but also include trainings, public outreach activities, educational materials, press releases, meetings, plans, expanded social networks, decision frameworks and decision support tools, etc.
C. Evaluation and Effectiveness:
What factors shape the evaluation of research?
Science-centric: Quantitative and statistical approaches are used to evaluate outputs and are consistent with a linear, “knowledge transfer” framework (Godin 2005; Best and Holmes 2010). Evaluation is most commonly performed through bibliometric analysis by quantifying the number of peer-reviewed publications, the impact factor of the journals where the papers are published, and the number of times the papers are cited. Newer methods include the “h-index” and network analysis of research collaborations, however these are not widely used by funding agencies to evaluate research productivity. Outcomes are not typically evaluated.
Public-value oriented: Evaluation includes the aforementioned approaches but also includes an “extended peer community” beyond the world of researchers (Funtowicz and Ravetz 1993). Using traditional bibliometric analyses alone limits our evaluation of these approaches and may even deter interdisciplinary research (Penfield et al. 2014; Rafols et al. 2012). Evaluating research productivity needs to include qualitative approaches using network and systems approaches to describe how knowledge is used in policy and the impacts it has on social values and preferences (Cozzens and Snoek 2010; Bozeman and Sarewitz 2011; Meyer 2011; Best and Holmes 2010; Donovan 2011). Producing, exchanging and integrating knowledge to support decisions is a complex process, thus the widest array of outcomes should be evaluated, including outcomes oriented to process and interactions between researchers and users (Fazey et al. 2014; Molas-Gallart et al. 2014; Moser 2009). Evaluating outcomes related to social capital, knowledge exchange, and influence is best served by the use of qualitative methods. Evaluating outcomes remains challenging due to long temporal delays between knowledge production, integration, and application, and because the effects of some decisions may not be able to be assessed for years afterward (e.g., flood mitigation planning or earthquake retrofitting).
D. Flexibility:
How easy is it to alter research to better respond to users’ needs, and changes in those needs?
Constrained: Research organizations are relatively inflexible with formal rules of operations. The inflexibility is not a problem given the rather predictable forms of research conduct and applications.
Responsive: Responding to users’ needs, emerging problems, and expanding research windows of opportunities requires higher degrees of organizational flexibility (McNie 2013).
E. Human Capital:
What kinds of skills and training are needed to do the research?
Narrow: Researchers are trained almost exclusively as Ph.D.s and have gone through rigorous training as doctoral and post-doctoral researchers.
Broad: Researchers have a broader array of skills, training, and experiences and may include people with master’s degrees or even individuals with expertise gained from lived experiences.
F. Boundary Management (the boundary between science and society needs to be managed to accomplish two mutual goals: ensuring that research responds to the needs of users and assuring the credibility of science; boundary work involves communicating between science and society, translating information, and mediating and negotiating across the boundary):
To what extent must efforts be made to actively manage the boundary?
Limited: The risk of politicization of science is low, although it varies by discipline, but increases somewhat with more applied research. Society, especially politically marginalized populations, has little or no voice in research. Thus, little attention to boundary management is necessary.
Broad: Active involvement of users in shaping research brings science and users closer together, inevitably increasing the risks of its credibility being impugned or of science becoming politicized. Such conditions will require greater efforts in boundary work by individuals and organizations.
IV Using the Typology
The typology provides a richer depiction of the character and conduct of science than permitted by terms like “basic” and “applied.” Such terms are often used with vague and varied definitions, often for political purposes in the competition for resources. Moreover, individual science projects, programs, and institutions change over time in response to many of the contextual factors mentioned in the attributes. For example, leaders of science institutions can have a dramatic effect, shifting the focus of an institution to or from particular goals or societal needs. Also, federally funded, national scale programs are being designed with the goal of building capacity to connect science with societal needs. But science managers lack methods to empirically assess whether this capacity is actually being achieved, a wholly different process than that of evaluation of impact, outcomes, or public value of an individual effort.
The typology provides a framework to visualize the range of activities of a science project or several projects across a program or institution at both fixed points in time and over a succession of years. To operationalize the use of the typology, one might allow the spectra to vary on a scale of one (science-centric values are dominant) to five (user-oriented values are dominant), and expert judgments can be applied to classify the full menu of attributes for a given project or set of projects (e.g. program).
Example 1: Southwest Monsoon Fire Decision Support
Wildfire suppression in the United States is built on a three-tiered system of geographic support: a local area, one of the 11 regional areas, and finally, the national level. When a fire is reported, the local agency and its firefighting partners respond. If the fire continues to grow, the agency can ask for help from its geographic area. When a geographic area has exhausted all its resources, it can turn to the National Interagency Coordination Center (NICC) at the National Interagency Fire Center (NIFC) for help in locating what is needed, from air tankers to radios to firefighting crews to incident management teams. Predictive Services was developed to provide decision-support information needed to be more proactive in anticipating significant fire activity and determining resource allocation needs. Predictive Services consists of three primary functions: fire weather, fire danger/fuels, and intelligence/resource status information. Predictive Services involves participation from representatives of the Bureau of Land Management, Bureau of Indian Affairs, National Park Service, Forest Service, U.S. Fish and Wildlife Service, Federal Emergency Management Administration, and the National Association of State Foresters. In this project, researchers from the Desert Research Institute (Reno, NV) and the University of Arizona identified needs within the Southwest Area, Rocky Mountain, and Great Basin Predictive Services information in relation to the Southwest Monsoon to better understand the physical relationships between monsoon atmospheric processes and fire activity. The results of the project will provide operational fire staff and managers with information and products to improve prediction of monsoon impacts on fire, and climatological information on monsoon-fire relationships for assessments and planning. Figure 2 represents the program manager’s classification of this research for each attribute, based on elements of the project description above and extensive interaction with the researchers on the project.
While the activities may be idealized, the example in Figure 2 illustrates how research projects are not idealized. If the Spectra of Research Criteria vary on a generic scale of 1 – 5, then a science manager could assign a score of 3 to Relevance, given that the monsoon information may not fit the decisions of both the Southwest and Great Basin. Similarly, a science manager could assign a score of 5 to Expertise, since the experience of fire managers and agency personnel help shape the decision support information. Moreover, this example illustrates how research directed toward satisfying the needs of decision makers need not have every attribute scored as a 5, which adequately reflects the complexity of research design and implementation.
Example 2: Indonesian Agroforestry
On the island of Sumatra, “protection forests” provide several economic and ecosystem services including erosion control, water filtration, and timber production. Agroforestry practices, such as coffee cultivation, were not allowed in protection forests limiting the opportunity for people to improve their livelihoods. The Ministry of Forestry believed that coffee trees were not “trees” in that they could not provide the same ecosystem services as timber species. In the interest of improving livelihoods for impoverished farmers living in the protection forests, the World Agroforestry Centre sought to determine if coffee trees were in fact trees in terms of providing similar ecosystem services as timber species. From a disciplinary perspective the research was fairly narrow, scoring a 2 on the spectra (See Figure 3). Research determined that coffee trees did in fact provide similar ecosystem services as timber varietals. This research was complex for many reasons. One is that findings from the research had implications in how protection forests were managed that could result in transferring some power for land management from the government to local tenant farmers. The World Agroforestry Centre was largely seen as a trusted broker of information, but significant mistrust existed between the government and farmers, consequently increasing the need for the research to be both credible and legitimate, and for managing the boundary between science and society thus scoring a 5 on the spectra.
In both examples, producing scientific information to inform decision making and improve societal outcomes was the goal. Yet each project had different characteristics including users, role of expertise and disciplinary foci, knowledge exchange, and others. Differences between approaches does not indicate that one is better than the other, but rather, that shaping research to meet the needs of users requires different approaches.
For the science manager, the most important element of putting the typology into practice through such graphical tools is in bringing to the fore aspects of the research process that are often unexamined, assumed, or excluded. Using the typology will create a process of developing and visualizing empirical evidence and expert judgments about the internal coherence of science projects, programs, and institutions. It will also allow deeper understanding of how they are evolving over time. For a program or institution, this exercise could be done for any number of projects to yield an aggregated sense of the type of research being performed. This process would allow science managers to: a) form mental models about mechanisms that might support different attributes and activities over time, b) dispel bias about whether or not the research is complementing mission and goals that support user-driven science, and c) link observed changes in the character and conduct of science to observations of contextual factors (e.g. a decrease in knowledge exchange resulting from a different project or program leader). The typology can aid in the development of proposal solicitations, in allocation decisions about resources to support research, and in devising more valuable grant-reporting processes. The tool still requires intimate familiarity with the research in question, which should be developed through extensive interaction with knowledge producers and users as partners in a collective endeavor. This task often requires the soft skills and expertise referenced in the Knowledge Exchange attribute.
For the science policy community as a whole, putting the typology to use over time can help bring the expert judgment of science managers to bear on understanding the relations among (a) the complex attributes of research activities, (b) the expectations for and promises about the goals of science, and (c) the extent to which research activities actually are appropriately structured to advance desired societal outcomes. Applying the typology, or other rigorous methods of science policy assessment, across broad portfolios of science can support responsible decision making about science that is justified by, and aimed at, the achievement of public values in addition to simple knowledge creation.
V Acknowledgements
We thank the David and Lucile Packard Foundation, and especially Kai Lee, for their support of this project.
VI Citations
Adler, P.S., Kwon, S.W. 2002. Social Capital: Prospects for a New Concept. The Academy of Management Review 27 (1): 17-40.
America Competes Act. 2007. H.R. 2272, 100th Congress, 2007-2009. Signed August 9, 2007.
Argyris, C. 1976. “Single-Loop and Double-Loop Models in Research on Decision Making.” Administrative Science Quarterly 21 (3): 363.
Averyt, K. 2010. Are we successfully adapting science to climate change? Bull. Amer. Meteor. Soc., 91: 723–726.
Berkes, F. 2009. Evolution of Co-Management: Role of Knowledge Generation, Bridging Organizations and Social Learning. Journal of Environmental Management 90 (5): 1692–1702.
Best, A. and B. Holmes. 2010. Systems Thinking, Knowledge and Action: Towards Better Models and Methods. Evidence & Policy: A Journal of Research, Debate and Practice 6 (2): 145–59.
Bozeman, B. and D. Sarewitz. 2011. Public Value Mapping and Science Policy Evaluation. Minerva 49 (1): 1–23.
Bush, V. 1945. Science: the Endless Frontier. Office of Scientific Research and Development. Washington DC.
Calvert, J. 2006. What’s Special about Basic Research? Science, Technology & Human Values 31 (2): 199–220.
Clark, W. C., T. P. Tomich, M. van Noordwijk, D. Guston, D. Catacutan, N. M. Dickson, and E. McNie. 2011. Boundary Work for Sustainable Development: Natural Resource Management at the Consultative Group on International Agricultural Research (CGIAR). Proceedings of the National Academy of Sciences, August. www.pnas.org/cgi/doi/10.1073/pnas.0900231108
Clark, W. C. and N. M. Dickson. 2003. Sustainability Science: The Emerging Research Program. Proceedings of the National Academy of Sciences 100 (14): 8059–61.
Cozzens, S. and M. Snoek. 2010. Knowledge to Policy: Contributing to the Measurement of Social, Health, and Environmental Benefits. White paper prepared for the “Workshop on the Science of Science Measurement”, Washington D.C. December 2-3, 39 pgs.
Dilling, L. and M.C. Lemos. 2011. Creating Usable Science: Opportunities and Constraints for Climate Knowledge Use and Their Implications for Science Policy. Global Environmental Change 21: 680-689.
Donovan, C. 2011. State of the Art in Assessing Research Impact: Introduction to a Special Issue. Research Evaluation 20 (3): 175–79.
Epstein, S. 1995. The Construction of Lay Expertise: AIDS Activism and the Forging of Credibility in the Reform of Clinical Trials. Science, Technology and Human Values. Vol 20 (4): 408-437.
Fazey, I., L. Bunse, J. Msika, M. Pinke, K. Preedy, A. C. Evely, E. Lambert, E. Hastings, S. Morris, and M. S. Reed. 2014. Evaluating Knowledge Exchange in Interdisciplinary and Multi-Stakeholder Research. Global Environmental Change 25 (March): 204–20.
Funtowicz, S. O., and J. R. Ravetz. 1993. Science for the Post-Normal Age. Futures 25 (7): 739–55.
Geels, F. W. 2004. From Sectoral Systems of Innovation to Socio-Technical Systems. Research Policy 33 (6-7): 897–920.
Godin, B. 2005. The Linear Model of Innovation: The Historical Construction of an Analytical Framework. Project on the History and Sociology of S&T Statistics. Working Paper No. 30. 36 pgs.
Guston, D.H. 2001. Boundary organizations in environmental policy and science: An introduction. Sci. Technol. Hum. Values 26 (4): 399–408.
Jasanoff, S. 2004. The idiom of co-production. In Jasanoff S (Ed) States of Knowledge: the co-production of science and social order. London, Routledge. 1-12.
Keen, M. and S. Mahanty. 2006. Learning in Sustainable Natural Resource Management: Challenges and Opportunities in the Pacific. Society and Natural Resources, 19 (6): 497-513.
Kirchhoff, C. J., M. C. Lemos, and S. Dessai. 2013. Actionable Knowledge for Environmental Decision Making: Broadening the Usability of Climate Science. Annual Review of Environment and Resources 38 (1): 393–414.
Knapp, C.N., Trainor, S.F. 2013. Adapting science to a warming world. Global Environ. Change 23: 1296-1306.
Knowles M.S., E. F. Holton III, and R. A. Swanson. 1998. The Adult Learner. Routledge: New York.
Latour, B. 1987. Science in Action: How to Follow Scientists and Engineers Through Society. Harvard University Press.
Latour, B. and S. Woolgar. 1979. Laboratory Life: The Construction of Science. Princeton University Press.
Lemos, M. C., C. J. Kirchhoff and V. Ramprasad. 2012. Narrowing the Climate Information Usability Gap. Nature Climate Change 2: 789-794.
Lemos, M.C. and B.J., Morehouse, 2005. The co-production of science and policy in integrated climate assessments. Global Environ. Change 15: 57–68.
Lempert, R., N. Nakicenovic, D. Sarewitz, and M. Schlesinger. 2004. Characterizing Climate-Change Uncertainties for Decision-Makers. An Editorial Essay. Climatic Change 65 (1): 1–9.
Levin, D. Z. and R. Cross. 2004. The Strength of Weak Ties You Can Trust: The Mediating Role of Trust in Effective Knowledge Transfer. Management Science, Vol. 50(11): 1477-1490.
Logar, N. 2009. Towards a Culture of Application: Science and Decision Making at the National Institute of Standards & Technology. Minerva 47(4): 345–66.
Lövbrand, E. 2011. Co-Producing European Climate Science and Policy: A Cautionary Note on the Making of Useful Knowledge. Science and Public Policy 38(2), 225-236.
McNie, E. C. 2013. Delivering Climate Services: Organizational Strategies and Approaches for Producing Useful Climate-Science Information. Weather Climate and Society 5: 14-26.
McNie, E. C. 2008. Co-Producing Useful Climate Science for Policy: Lessons from the RISA Program. Monograph, University of Colorado, Boulder.
Merton, R. K. 1945. Role of the intellectual in public bureaucracy. Social Forces 23 (4): 405–415.
Meyer, R. 2011. The Public Values Failures of Climate Science in the U.S. Minerva 49 (1): 47-70.
Mezirow, J. 1997. Transformative Learning: Theory and Practice. New Directions for Adult and Continuing Education. No. 74. San Francisco: Jossey-Bass.
Michaels, S. 2009. Matching Knowledge Brokering Strategies to Environmental Policy Problems and Settings. Environmental Science & Policy 12 (7): 994–1011.
Molas-Gallart, J., P. D’Este, Ó. Llopis, and I. Rafols. 2014. Towards an Alternative Framework for the Evaluation of Translational Research Initiatives. INGENIO (CSIC-UPV). http://www.ingenio.upv.es/sites/default/files/working-paper/2014-03.pdf.
Moser, S. 2009. Making a Difference on the Ground: The Challenge of Demonstrating the Effectiveness of Decision Support. Climatic Change 95 (1-2): 11–21.
NRC – (National Research Council). 2005. Decision Making for the Environment: Social and Behavioral Science Research Priorities. National Academies Press, Washington, DC.
NRC (National Research Council). 2009. Informing Decisions in a Changing Climate—Panel on Strategies and Methods for Climate-Related Decision Support. National Academies Press, Washington, DC.
NRC (National Research Council). 2010. Informing an Effective Response to Climate Change. National Academies Press, Washington, DC.
NSB (National Science Board), 2010. Key Science and Engineering Indicators: 2010 Digest. Roesel, C., Ed. NSB 10-02. National Science Foundation.
Nonaka, I. 1994. A Dynamic Theory of Organizational Knowledge Creation. Organization Science 5 (1): 14–37.
Nowotny, H., P. Scott and M. Gibbons. 2002. Re-Thinking Science: Knowledge and the Public in an Age of Uncertainty. London: Polity Press. 278 pgs.
OECD – Organisation for Economic Co-operation and Development. 2002. Frascati Manual: Proposed Standard Practice for Surveys on Research and Experimental Development. 254 pgs.
Pahl-Wostl, C. 2009. A Conceptual Framework for Analysing Adaptive Capacity and Multi-Level Learning Processes in Resource Governance Regimes. Global Environmental Change 19 (3): 354–65.
Penfield, T., M. J. Baker, R. Scoble, and M. C. Wykes. 2014. Assessment, Evaluations, and Definitions of Research Impact: A Review. Research Evaluation 23 (1): 21–32.
Polanyi, M. 1962. The Republic of Science. Minerva 1: 54-73.
Rafols, I., L. Leydesdorff, A. O’Hare, P. Nightingale and A. Stirling. 2012. How journal rankings can suppress interdisciplinarity. The case of innovation studies and business and management. Research Policy 41(7): 1262–1282.
Sarewitz, D. 2004. How Science Makes Environmental Controversies Worse. Environmental Science & Policy 7(5): 385–403.
Sarewitz, D. and R. A. Pielke. 2007. The Neglected Heart of Science Policy: Reconciling Supply of and Demand for Science. Environmental Science & Policy 10 (1): 5–16.
Stokes, D. E. 1997. Pasteur’s Quadrant: Basic Science and Technological Innovation. Brookings Institution Press: Washington, DC.
Trist, E. 1981. The Evolution of Socio-Technical Systems: A Conceptual Framework and an Action Research Program. Occasional Paper No. 2. June. York University, Toronto. 67 pgs.
Van den Hove, S. 2007. A Rationale for Science–policy Interfaces. Futures 39 (7): 807–26.
Ziegler, R. and K. Ott. 2011. The Quality of Sustainability Science: A Philosophical Perspective. Sustainability: Science, Practice, & Policy 7 (1): 31–44.